Data-Driven Project Management: Best Practices for Beginners
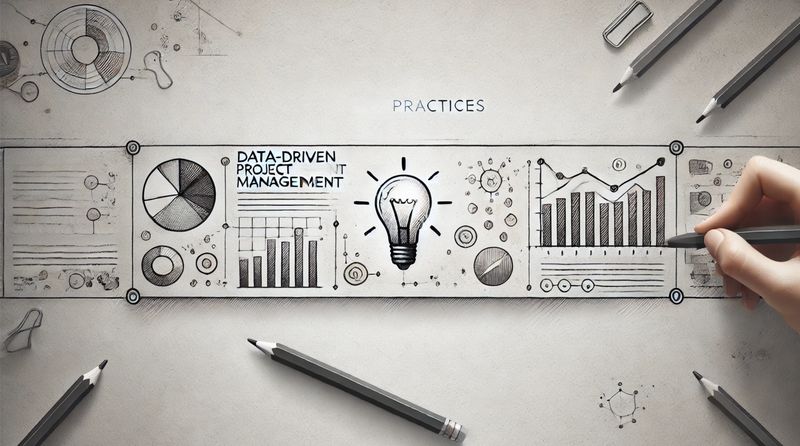
Setting Up a Data-Driven Foundation
The first step in data-driven project management is establishing a strong foundation to ensure you’re capturing, organizing, and interpreting the right data. A great place to begin is by clearly defining the project goals and key performance indicators (KPIs). Knowing what you want to measure makes it easier to collect meaningful data and track progress effectively.
To define your KPIs, start with project objectives like reducing project costs, improving timelines, or enhancing customer satisfaction. If your goal is to complete a project under budget, then KPIs could include the average cost per task or time spent on specific phases. For another approach, you could use time-to-completion data to understand where you might cut down timelines without sacrificing quality.
Next, set up a centralized data tracking system to organize and store all project data in one place. Tools like project management software (e.g., Asana, Jira, or Monday.com) offer integrated data dashboards, allowing you to track project KPIs, monitor team progress, and even automate routine reports. For a hands-on approach, consider software with customizable fields so you can tailor data tracking directly to your project’s needs. When your data is easily accessible, it’s simpler to spot trends, make adjustments, and keep stakeholders informed.
Analyzing Data for Decision-Making
Once you’ve established a foundation, effective data analysis is essential for making informed project decisions. Start by learning to interpret the data you’ve collected in relation to your KPIs and project objectives. For example, if you notice increasing task completion times, this may signal that your team is overburdened or facing inefficiencies in a particular phase. By identifying the source of delays, you can introduce targeted improvements, like reallocating resources or providing additional training.
Another approach to data analysis is comparing your data over time to spot trends that can guide future decisions. For instance, if previous projects consistently faced delays during the testing phase, you could adjust timelines or bring in additional support for that stage in new projects. Additionally, consider using predictive analytics if your project management software includes this feature. Predictive tools can help forecast future delays or cost overruns, allowing you to adjust plans before issues arise. Such tools might show, for example, that certain teams often experience increased workloads near project deadlines, helping you adjust schedules proactively.
Finally, be sure to validate your findings by discussing data insights with your team. Even the most thorough analysis can benefit from additional perspectives, and team feedback can help clarify if certain metrics reflect reality. For instance, if data suggests a specific resource is underused, a team member might reveal that this resource is actually crucial but used infrequently. This collaborative approach ensures that your data-backed decisions are accurate and actionable.
Communicating Data-Driven Insights to Stakeholders
Effectively communicating insights derived from data is just as important as gathering and analyzing the data itself. Begin by translating complex metrics into clear, straightforward language that all stakeholders can understand. For example, instead of discussing “variance in time-per-task across phases,” say “the design phase took 10% longer than planned, so we’re reallocating resources to keep on track.”
When presenting data to stakeholders, focus on how insights impact the project’s overall goals. If a specific KPI has improved, explain how it aligns with broader objectives, such as delivering the project ahead of schedule or staying under budget. Using a narrative format can help illustrate how each data point contributes to the story of the project. For instance, you might explain that by reallocating resources based on time-to-completion data, the project’s final phase was finished earlier than expected, which allowed for additional quality assurance checks.
For data visualization, use simple, intuitive charts or graphs to make your insights easily digestible. Tools like Power BI or Tableau offer interactive dashboards where stakeholders can explore the data further if they wish. Visual aids help provide context, making it easier for non-technical stakeholders to grasp trends and conclusions. When choosing a visualization, opt for a bar graph to compare performance across different tasks, or use line charts to show trends over time. Visualizations should always support the key points you’re conveying, reinforcing the narrative of how data supports project success.
Finally, encourage two-way communication by inviting questions and feedback from stakeholders on your data insights. When stakeholders feel involved, they are more likely to support data-driven decisions. This collaboration can even reveal additional insights, as stakeholders might interpret data from a unique perspective.
In summary, data-driven project management empowers project managers to make informed, objective choices that directly benefit project outcomes. By setting a solid foundation, focusing on meaningful analysis, and communicating clearly with stakeholders, you can leverage data to enhance your decision-making and ultimately achieve project success.